Navigating the New Tech Landscape
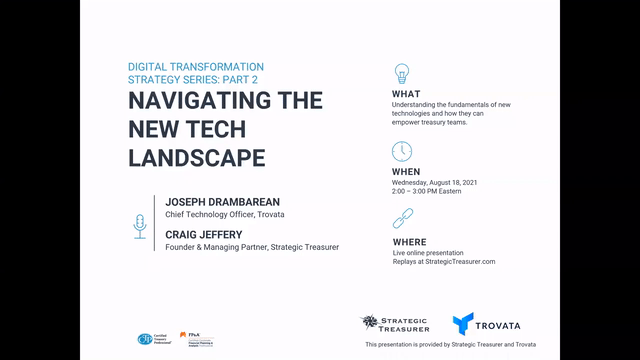
Visibility, data connectivity and forecasting are crucial to staying afloat in today’s climate, and leveraging new technology is becoming more and more crucial to achieving those goals. From RPA to AI and ML, the alphabet soup is confusing but increasingly important to treasury’s daily life. Master the fundamentals of these technologies and how they can empower your treasury team to unlock efficiency and access accurate information.
Ky: All right. Hello and welcome to today’s webinar on navigating the new tech landscape! This is Ky from Strategic Treasurer. And I’m glad you could join us for the second webinar in the Digital Transformation Strategy series. Before I introduce today’s speakers, I have just a few quick announcements. The Zoom platform allows for several different ways to interact today. One is a chat available by using the chat box icon on your toolbar. So you can post comments and/or questions viewable by all attendees. If you’d like to ask your question to just the presenters, please use the Q&A icon on the toolbar. This is a full-hour presentation. And we encourage you to ask your questions at any time during the presentation, and we’ll try to get to as many as we can. If we can’t get to all the questions, someone from our team will follow up with you. There will also be a couple of polling questions throughout today’s webinar that you’ll be able to select your response from a list of multiple choices. If you are viewing on multiple screens today, the poll question might appear on a different screen than the presentation. There’s also an added step in this platform that’s different from our past polling questions. You will need to hit the “Submit” button to have your response recorded. All right, our presenters today are Joseph Drambarean, Chief Technology Officer at Trovata, and Craig Jeffery, Founder and Managing Partner at Strategic Treasurer. Welcome, Craig and Joseph! I’ll now turn the presentation over to you.
Craig Jeffery: Thank you, Ky! Welcome, everyone! I’m glad you could join us for today’s webinar. I wanna say hello to Joseph. I’m glad to be talking about technology with the chief technology officer. Welcome, Joseph!
Joseph Drambarean: Yeah, thank you so much! I’m really excited about our conversation today. We’re gonna cover so many topics.
Craig Jeffery: Yeah, but it should be excellent. And Ky gave you, everybody, a lot of info about how we can stay connected on today’s session. So those chat boxes make perfect sense for questions as well as the Q&A. I’m certainly open to being connected on LinkedIn if you’d like to connect that way. And there are other ways we can stay connected separately as well. We’ll drop those into the chat box throughout the session.
So let’s get started with today’s overview. So this is our agenda. We’re gonna talk through these sections roughly in this pattern. So data history is the idea of what’s been changing with data, how rich is it, how much is there, and how that has impacted technology generally, but also how this evolution and revolution of data is impacting treasury.
We’ll look at the concepts of usable infrastructure. And this is the idea that the more modern technologies that exist allow us to work farther into the future to prepare for the long haul, to work faster, more responsive, and then more dynamic. And so this is the concept of, “How do we build in a way that we’re building and not throwing things out and rebuilding every year, every couple of years?” So this is an infrastructure that makes sense with the new tech.
Then, we’ll look at the needed mindset. And when we think about the concept of a mindset, it’s how we think. And so the subtext there is, “How do we think about next-gen processing, next-generation processing?” And some of the terms you’ll hear Joseph talking about in the dialogue we’ll cover, it has to do with scale, speed, a platform mindset, and how this has an impact on treasury activities, not just technology but on the business of treasury.
And then, in the other one, we’ve got a little red robot that says, “Beyond Human Insights”. Don’t worry! There’s plenty of jobs for us humans. But we’ll talk about deep learning. And the idea of some of the tools that can be employed, that are employed can make a significant difference and move us beyond what’s possible, using our brains alone because we can scale with computer technology. Just like you can dig with your hand, you can dig with a shovel, you can dig with a bobcat, you can dig with some giant earthmover equipment. And each of those, once you get past the shovel, you’re moving days, weeks, or months worth of activity that you could do without those tools. And so how do we leverage those tools that go beyond human physical capacity, but also beyond human insights? And so that’s the analog to the digital world.
And we’ll spend some time on the impact on treasury. And so it’s great to talk about technology. It’s also great to discuss our mental readiness and our technological readiness. And so we’ll see how this applies to what we do, how we think about it, how we develop our teams.
And then finally, there are a few thoughts at the end that wrap up some of the concepts, ideas, and facts that we’ll be discussing today.
So with that, we’ll move over to the history of data. And I’ll give a few intro words, and Joseph will comment or argue about what we’ve talked about. So let’s look at what we see here. We have a history of data, a few images here. File-based to API. And we’ll talk about that detail of creating files, sending them versus real-time or streaming activity. And Joseph has a number of comments throughout today’s session. We’ll also look at how data has changed from we couldn’t send it very quickly… It was very costly to send it, so everything was compressed. Everything was stripped out to enhance metadata. What is that? How is data smarter and more rich? What does that mean? And then, there are some of the concepts of unstructured and structured.
But before I get Joseph involved here, think about the amount of data that’s growing. We see 40 to 60% growth rates in the total data that we have. Whether it’s data that’s exported from systems that we have, from the Internet of Things, from capturing data in different places, data is essentially doubling every two years. And when you think about, “What does that look like over a decade?”, that’s two to the fifth. And as those of you who love math know that’s 32 times as much data will exist in 10 years than it does today. And vice versa, the current data that we have today is… We only had about 3% of the total data in the past. That’s a completely transformed way of looking at data. We have more data. We’re perhaps overwhelmed by data. But Joseph, as you’ve…
Joseph Drambarean: Yeah!
Craig Jeffery: …looked at this, I’d love for you to weigh in.
Joseph Drambarean: It’s actually really difficult to avoid the temptation of doing a full dissertation on the history of computers when looking at a slide like this. But I mean, the real context here is in the fact that over the last three decades, if you want to look at it at a macro view, there have been improvements not only to computers themselves but also bandwidth. And because of those improvements, whether it’s going from dial-up to the modern-day, if you want to call it that, high speed, and then from high speed to fiber, and then fiber to whatever will be the future, each step change is ultimately a throughput step change. And a lot of the formats, not just within banking but throughout the rest of the business world, they were defined 20-30 years ago for storing data in a way that’s organized and takes advantage of large Oracle databases, things of that nature. Those data sets were based on an assumption that you wouldn’t be able to send large amounts of data through the interwebs efficiently. And as a result, there were all kinds of buffers set in place, truncations, things that limited the nodal relationships of that data from being exposed to the end data consumer. And that’s what’s happening when we’re talking about the transition to a more API-based approach or a streaming-based approach from a data perspective.
Those limitations that would have existed and were very real from a hardware perspective 10-20 years ago are now starting to massively dissipate. And the conversion from those databases to ones that are more metadata rich, ones that are able to create these nodal relationships through graph networks, that is driving this ability, this newfound ability of having enhanced metadata. That metadata ultimately is useful for understanding the relationships and the context behind an individual transaction or an individual event, which is why planning for a future that takes advantage of metadata is so important. It’s because a transaction isn’t just as simple as its identifier and how much money was transacted. There are all kinds of context that live underneath it, whether it’s a payment or remittance information, or whether it’s information that could be coming from your ERP system or a different platform that provides context, that direction around, “How did this transaction occur? Why did it occur? Was it a transaction that is fraudulent for whatever reason? And how would you even establish that in the first place without doing a manual intervention?”
Well, the transition to having the nodal relationships that you would need in your data structure to get to a point of analyzing that individual record and knowing this record is related to all of these other records, and in its kind of totality, it represents this event having taken place, that is kind of what we think about when we think about this big transition, this transition of taking many fragmented data sources, whether it’s payment systems, or operational systems, or sales systems, or ERP systems, all of which have important contextual data that end up being resolved into a cash transaction. And while that cash transaction might be the end-all, the proof of whatever that event was, that data trail is really important because that data trail establishes lineage, it establishes important context. And as you define these more modern approaches to data infrastructure, it has to be done with this consideration in mind, that the end record might not be the totality of the history. It might be the tip of the spear, the top of the iceberg if you will. And as you have a more broad view of those nodal relationships, you inform the downstream processing that may take place, whether it’s headless machine learning models that are trying to intuit some sort of context that could lead to an insight, whether it’s processing that’s trying to identify fraud or trying to identify anomalies. All of these disparate pieces of data inform those models and give the context needed to make an accurate programmatic decision, one that would replace hours and hours of human data transformation, normalization, and ultimately, then analysis to drive the same insight. So it all starts with the data.
And because the history of the data that we are using today has its roots so far back in the limitations that were imposed based on hardware and bandwidth requirements of the day, there’s really this more meta-level of data transformation that is also taking place. It’s the “How far can we take the context of a record?” and “How wide can those nodal relationships be established?” so that ultimately when you’re looking at a point in time record of a transaction or something that took place financially, you can see all of its related metadata. And that’s really kind of the foundation of a lot of the things that we’re talking about today. It’s the depth of your data.
But there’s this other piece that I haven’t really touched on, which is the speed of data, right? So in the past, the bandwidth limits were primarily from a data structure and the amount of data that you could get. There is also a time component to it. Maybe, you were not able to query as often because of duress that might be placed on the system because of an on-prem installation or on-prem hosting. And maybe, you only paid for so much server capacity to be able to handle those kinds of operations. And this happens today, whether it’s within the enterprise, whether it’s within the bank, whether it’s within even tech companies. It’s that old-school approach to cloud computing and whether or not you can take advantage of elastic scale and elasticity overall with regards to compute. As data transformation has continued to percolate together kind of hand-in-hand with cloud transformation, what we have seen is the availability of compute and availability of bandwidth that allows for you to do things in a more real-time way. And instead of having to place job requirements and cron out your schedule of when and why you’re going to get the data, now the expectation is, “I want it now,” and even better, “I want it proactively. I just wanna be made aware when there’s new data.” This model of interactivity is fundamentally different from one that is based on an active query. And that’s something that needs to be kept in mind when thinking about how far we’ve come. We’ve come from a place where you had to get time on a terminal to get access to a database so that you could get access to compute. Now, there’s no such concept. I mean, it is literally a network of individual virtualized servers that can be something at any time, whether you’re in AWS or Google Cloud or Microsoft Azure. Their resources are not only on demand, but it’s also globally available, which is quite the step change.
Craig Jeffery: Yeah, I think you laid out a number of things. Like think about, “Oh, we’re gonna talk to our grandchildren and say, “We had a stick and memory chips ourselves to scale up,” and they’re like, “What do you mean? We just provision those.” But let me ask you just a couple of things, Joseph, on this row. I’ll try to touch on two of these items. When we talk about file-based versus API, maybe, you’ll like this analogy, maybe you don’t, I think a file-based is… Let’s say, you wanted water in your house, and there was a well down the road, and you’d go down with a bucket, you’d fill it, you’d bring it back, and you’d pour it in the sink or your bath or whatever you do. That’s the analogy or what’s analogous to file-based. API is you have water, a huge pipe, high pressure running to your house that distributes it to your refrigerator, your sink, etc. It’s when you need it. It’s there. Is that it?
Joseph Drambarean: Right! That’s an excellent analogy because it actually is an easy way to grasp the difference between something that is a prepared output versus direct access. An API ultimately is programmatic access to an end resource, right? That’s an application programming interface. That’s what API stands for. And what you get when you have an API is ultimately a way to access the end resource directly. And that resource might make certain things available from itself: certain data types, certain capabilities, certain date ranges, etc. And it gives you the ability, as the end user, to take advantage of those different capabilities and get the data that you might need.
A file is ultimately a prepared object. It’s a report. It’s a thing that was extracted from that same database that we might be operating from. But instead of you having any control over how and why you might wanna take data out, you have to work within the constraints of, “There is some widget somewhere on the backend that is preparing that file for you and then sending it wherever you want it to be sent.” And that is the extent of customization that you might have after the fact.
And ultimately, that’s really the difference. It’s the difference between a bucket, like you said going to the well, grabbing a full bucket of water, and pouring it into the sink, or taking a hose and connecting it all the way into the well and getting that water as fast as you want it or as much as you want at any time. And it changes your approach altogether because of how you can get the data for various use cases and various reasons. Whereas in the file-based approach, you have to create a universe of functionality within that one file and hope and pray that everyone downstream that will need that information has everything that they need within that file.
Craig Jeffery: The one other thing I wanted to just talk on real briefly before we move ahead… You used a lot of different terms that were useful. But from a level-set perspective, the limited data and enhanced metadata… I know this won’t do it full justice. But limited data… We talked about past data. It used to be extremely expensive to send data. And so they stripped everything out. They delimited it, they put commas between fields. And so the date would be in position three, and the transaction amount would be in position four. And it was all…
Joseph Drambarean: Right!
Craig Jeffery: …determined by location. And so then, you have different types of metadata or Extensible Markup data. So now, if the data is moving, it comes with… This is an amount, but it comes with a package that says, “This is the transaction amount,” with it.
Joseph Drambarean: Right!
Craig Jeffery: And that’s the type of metadata. Is that enough for our discussion today? Or do we need to think about metadata more broadly?
Joseph Drambarean: Yeah, I think that’s a starting point, for sure. But another aspect of metadata that’s important is nested relationships within that metadata. So if we’re thinking about it… I’m tossing a technical term out there. But when you think about a JSON structure for a payload of data, there’s a lot more flexibility with how you can create hierarchical classifications that may lead to important distinctions in your data. Just to give an example, maybe there is a parent record for an ACH, and that ACH is representative of 1,000 items, 1,000 individual payments that were swept under that ACH in a batch. And each one of those 1,000 items within it might have its own nested information, like individual remittance information for that payment, that in and of itself might also have nested information like the context of an address, or a payee, or identifiers, whatever it might be. That kind of laddered hierarchy is way more cleanly described in modern Object Notation than in… Take your example of a character-limited file that has to not only perform truncations but might have special characters that refer to a key somewhere in another file that expand that data with predefined terms, right? Like, for example, maybe the BAI code schema that each number represents a code, that code represents a certain type of transaction, etc., etc. So these different file types have that working against them or for them, depending on how you look at it and the fact that there’s an expertise that is required to even read the file in the first place. Now, if a machine is reading it, obviously, it infers all that information and can expound on it. But ultimately, you’re limited to whatever was written in that specification 20 years ago. So that’s not gonna change. Whereas in a more modern maybe API-based approach, you can version that API, you can say, “Between version 3 and version 4 of that API, in version 4, we now have a new data model. That new data model has 18 new fields, and those new fields have this context and this information.” And you can control which data you’re getting from which API, and it just gives you tremendous flexibility.
Craig Jeffery: Yeah, I think there’s a lot of good thinking too, good thinking around those comments that you have. I think there’s another part of that, too. It’s that also the older formats may be delimited. And if you stuck additional data in, they would break because the positions would be thrown off. It would create an inflexible future structure.
Now, as we shift to data foundations and capacity… And for those who’ve been submitting questions, we see those, we’ll take those in a moment. So feel free to keep popping those in. But I wanted to say a few things here on the data foundations. And some of this has been covered. But this is the idea of, there’s capacity technology that allows us to compute and scale up as opposed to being restricted physically. So the scale is a key component. The same thing with data. And we’ve spoken quite a bit on data: Where is it? How do we access it? How do we handle the volume of activity and continual acceleration of the amount of data that could be useful to us? This requires some type of infrastructure to support data capacity to compute, capacity to store and access.
Joseph Drambarean: And I love this slide. I think that it’s a great way to think about, almost if you will, step zero of making a data transformation strategy. It’s kind of starting at the foundation of, “What ultimately is this data going to do? And how do we need to accomplish the right infrastructure for taking advantage of those use cases?” And it’s not as simple as just finding a solution, if you will, going into the market and saying, “Okay, well, this checks the box on functionality that I want.” Maybe, the short term will solve a problem. But that long term, if it’s not flexible, it may limit your options with regards to future use cases that maybe are more advanced from an analytical perspective or from a data science perspective. And that’s why I think we’ve broken it into these two pieces, which are really representative.
Technology really, this is around the planning for the use case. So computers are a great example. When you think about the kinds of processes you will want to run, is it a scale problem? Is it one where you are taking advantage of thousands or hundreds of thousands, millions of records and having to correlate work through them quickly and find anomalies or find matches or reconcile that data? And how can you set up the right types of essentially server provisioning to take advantage of that kind of scale while also taking advantage of cost controls to be able to do it efficiently? Analysis, that is another branch of that in how you can take advantage of distributed server capacity to be able to analyze large cohorts of your data in isolation and be able to do separate functions without tripping up the main application. An interesting concept that has emerged really over the last 10 years is moving away from monolithic applications, single-purpose applications that do everything in one box to more distributed micro service-oriented applications that maybe are serverless and that are on-demand and available to do their compute time only when you need it and are not running in a way where it could create duress from a spend perspective and things of that nature. Those are important factors to consider. And it really comes down to, “What are you trying to do with this data?” Are you trying to plan for a future that is intensive in terms of processing, where you’re replacing ultimately human capacity with computer capacity to comb through that data, find important nuances in that data, find insights, find anomalies, and drive value from an end perspective that may impact multiple organizations, maybe not just the treasury organization? But really it all starts with data foundation again. It’s how you store that data.
There’s a lot of questions that have come up. When we have talked about it here, on the Trovata side, it comes down to a few key areas: findability of data, performance of the database (Ultimately, what kind of querying capacity are you trying to drive, milliseconds of performance? Are you trying to run these things on an operational level where maybe performance is not that important to the end-all?), and then, ultimately, richness. Can you take advantage of those nodal relationships that we were talking about? Are you able to store that data in a way where references are easily accessible? And even though maybe you have your root of a transaction record or a balance record or an account record, can you then infer all of its relationships quickly and efficiently and take advantage of search, take advantage of filtering, take advantage of easy findability? That ultimately comes down to database design and choosing the right database technology to give you those results because it’s not as simple as taking your data, putting it into a relational database, and then slapping SQL interface on top. If it were that simple, then we wouldn’t have data transformation as a problem.
Craig Jeffery: Excellent! So we’ve covered a lot of good information about data and technology, which is a foundation for much of what we’re covering. That brings us to our first poll question. And you’ll see that appear somewhere on one of your screens. Maybe, in the front, maybe in the back. This is a “Select all that apply”. What are you using for cash forecasting? So go ahead and select any or all of those items. While you’re doing that, there’s a couple of questions that came in. I’ll take a quick shot to answer a few of those, Joseph, and then we’ll comment on the cash forecasting piece.
One question is, “Are banks charging per transaction each time an API data is pulled?” That can vary. A lot of, I call it, more sophisticated banks charge the same or roughly the same currently for any way you pull the data. So they’ve neutralized the cost. And that may change over time, but that’s roughly what it is. The other question is, “Why would a company with a large data infrastructure in place, for example, Swift, abandon it and switch to APIs?” And so that’s a long answer. But Swift is changing from a messaging service to a platform, adding API capability and making shifts as well. But the one common is, we will talk about it later, it’s this idea of the use of infrastructure, the infrastructure that you build to the future. You don’t necessarily rip everything out instantaneously. And what do you replace when you replace older legacy formats? What’s the order of priority of those? Those are fairly thoughtful questions that require scale. You can’t do everything instantaneously. And so those are a couple of items.
But let’s look at the results. And we’ve got more items to include. Excel, number one. It doubles up a treasury system. And then, people are using ledger paper. I know some people type the messages that they wanted to answer that because of… That was my sense of humor. Not currently forecasting, 12%, Joseph. What do you think about these numbers?
Joseph Drambarean: I don’t think that Excel is surprising at all.
Craig Jeffery: No.
Joseph Drambarean: I think that I could have probably guessed that one in my sleep.
Craig Jeffery: Or 80%, yeah.
Joseph Drambarean: And ultimately, Excel is still the king of flexibility. And it’s extremely unlikely to be displaced in the next decade. But what we find when we use Excel, and as a daily user of Excel myself and Google Sheets and other spreadsheet tools, it’s ultimately the curation of that data and then the management of that data. Excel is great at dealing with ready-prepared data. It’s not great at doing it for you. In fact, it doesn’t do it for you at all. If that can be solved by Microsoft in the future, I think they will solve world hunger. But as of today, it doesn’t. And that’s why there are specialized tools that have emerged to try to fill in that problem space. And ultimately, we keep drawing these conclusions back to, “It really is all based on the data, and getting access to that data in a way that is normalized, in a way that is consistent, in a way that is performant so that you can ultimately do your post-processing, which is ultimately your analysis.”
And it’s interesting. When we think about this from an end application perspective because it’s easy to say, “Well, I should be able to forecast. Why can’t I just connect to all of these data sources and just forecast off of them?”, the reason is, ultimately, context. It’s that these different data sources mean different things and have ultimately different implications into your forecast. And so it’s not just a matter of aggregating that data into a commonplace and normalizing it. It’s then taking advantage of all of those disparate data types in a way that is sensible within the context of forecasting. So just to give you an example, cash transactions might be appropriate proxies for historical trends but might not be an exact science when it comes to predicting likely outcomes over the next year. AR and AP information coming from an ERP system might be a very precise science to a certain extent but may not be comprehensive in terms of your strategy of where things will go over the next year or two years. Sales data might fill in some of those gaps but might be really difficult to calibrate on because of the moving target that is a sales quota. All of these pieces together need to be treated in a way that takes advantage of the knowledge and the insight that is coming from the end data source but then creates a holistic system to ultimately visualize what you’re trying to visualize, which is a projection. And I think that it starts at the strategy level. It starts with planning out what you want to accomplish and then looking at your partners, looking at your data infrastructure, looking at your tools, and understanding where the gaps might lie. And it really comes from an understanding of that core data. And at Trovata, we obviously treat this with utter respect when it comes to trying to drive these different disparate data sets and taking advantage of them in a way that’s sensible. But really, it would apply for any use case, any tool, whether it’s even in Excel. If you’re trying to make a model in Excel, the normalization of that data, the context of that data, and the weights that apply to the model that you’re putting into your Excel workbook, how that data is refreshed, how often, and what it means every time you refresh it, all of that drives an accurate picture of where your forecast is going.
And when you think about it from a next-gen perspective, if you’ve solved the base problems of, “I know what type of data I’m working with. I know that it’s consistent, and I know that I can get it in a clean way,” then the next-gen is, “Can I do it on the fly? Can I do it in a way that is winsome and allows for me to explore and take advantage of post-processing and driving into a mode where, instead of being reactive and taking historical information and trying to work its way into something that can be modeled out, can I play, can I be in a strategic posture and explore different avenues, whether it’s more conservative outlooks or more aggressive outlooks, and be able to pull different knobs in a way that’s meaningful and that takes the data that I have and uses it as the context to drive all of that insight? And that’s really what it means to have a proactive forecasting mindset, one that can take advantage of your data as a platform, not as a database.
Craig Jeffery: Yeah, so Joseph, in terms of some of the things that we’ve covered, this data foundations mindset, I’m gonna leave this one without comments. It’s really moving “from” and “to”, and we’ve touched on a few of those. We’re not gonna get into these because I wanted to spend… As we look at the rest of the session, we have to step lightly on a few of these. So data tools. The first one is at scale. This is the bigger shovel. So I’d love it if you could talk particularly about the first bullet and then the last one. And I know we’re gonna get into the headless, serverless, deep learning…
Joseph Drambarean: Right!
Craig Jeffery: …supervised, unsupervised. So maybe, you can make a couple of comments about the at scale and deep learning.
Joseph Drambarean: Some of the most interesting data challenges that we’ve seen have been ones where the data exists and it’s fragmented and folks that are tasked with dealing with that data, normalizing it, collating it, turning it into reports, they just can’t keep up. They can’t keep up with the amount of data being brought in on a daily basis. And you can imagine it in industries such as e-commerce and payments and industries that deal with the enormous volume. And the volume has implications, implications to vendor payout, implications to reconciliation, implications to fees. And being able to take advantage of these structures and deals that you’ve set with the end consumer… Real-time processing and being able to take advantage of all of your data at the same time is a scale problem. It’s one that cannot be accomplished by throwing human bodies at it, especially when you think about some of the e-commerce companies that we have today. And so step one is just being able to keep up. And it turns out computers are great at that. Computers are great at being able to take a lot of disparate information, organize it, classify it, keep an eye on it, and then tell you at a point in time or proactively, “Hey, this is the latest and greatest information you need to know about where we stand on our bond payments. Here’s the latest and greatest information that you need to know in terms of how much our forecasted cash balance should be as of this date if you want to be able to make vendor payout.” These concepts are scale concepts. And they really play into the natural capabilities of cloud infrastructure being able to elastically expand to be able to process all of these different data types all at the same time and in a meaningful way, in a way that would give you that information at a moment’s notice so that you can make a decision off of that.
But then, one layer deeper is that machine doing it non-stop, right? In the past, maybe you were or a team was tasked with doing a report on an end-of-week basis or an end-of-month basis, and that report would define your strategy, right? It would define the insight that you need to be able to make a business decision. The world completely changes when the reporting is happening non-stop. And the insights that you would be garnering from that kind of technology would potentially drive more nuanced business decisions, first of all, but also more proactive business decisions. And that’s where taking advantage of machine learning comes into play because not only can you process that depth of information on the fly and on a real-time basis, but you can also have almost an “I have your back” mentality when it comes to defining how this technology works for you. Instead of you being the one that is telling the technology, “I need this. Give me something back,” the technology is saying to you based on your habits, based on things you have needed in the past, based on reports that have been created in the past, “We see these deltas. We see these insights. We see these anomalies.” And what’s great about that approach is that it really puts you in a posture of strategy, in a posture of leadership. Instead of having to work with your data, your data works for you and drives value in a way that gives you the context that you would need to make a business decision.
But what’s exciting is that there’s a whole other part of data exploration that we are still tapping into when it comes to ML and AI capabilities specifically, and that’s this deep learning sector. It’s the types of analyses and clustering techniques that you wouldn’t even have the inspiration to find if you were trying to do it yourself. You would need days and days if not weeks and weeks of focus in order to arrive at the conclusions that, what we call it, an unsupervised model might discover. And what’s great about using model-based deep learning approaches on data that is as explicitly typed as transactions is that a machine will find those groups organically. It will find those interesting intersections of different variables that you may not have found because of a variety of reasons. One could be a lack of focus. Another could be a lack of intent. Maybe, you were looking for something specific, but you don’t have the requisite intent to find anything else because it’s such a large task to find that original insight that you were looking for. Whereas a machine doesn’t have those limitations. It can organically explore every nook and cranny of your data across all of the various data types. So the metadata that we were talking about just a moment ago, this is really where you see the value. You see the value because the more relationships there are established in your transaction records, in your account relationships, in how those accounts play into different flows of transactions, whether it’s for payments, whether it’s for different operational needs. Those ultimately feed the possible intersections that would drive a deep learning model to discover an insight. And that’s where things get really exciting because it’s ultimately the DNA of your business that is uncovered and that is made available and displayed back to you to take advantage of it and use for further iteration, further exploration, further modeling, and ultimately further forecasting and business decision.
Craig Jeffery: Yeah, now I’m gonna summarize simply some of what you said just to tie in some of the words and phrases that we’ve been using today. So on the left-hand side, the at scale. This is being able to apply more processors to it. You used the phrase, “We can’t just throw more bodies at it.” When we move over to the deep learning side, some are the concepts about supervised and unsupervised. Supervised is you’re sending the system off to do tasks and functions in a more guided manner. Unsupervised, I think, is also what you referred to as “headless”…
Joseph Drambarean: Right!
Craig Jeffery: …where you’re throwing headless bodies at it, smarter tools to pull it. And those two things pull together. I’m going to move us to our next poll question that’ll pop up on your screen. There are two questions embedded. One is, “Which of the following is more significant?” You might need to expand it. And then, you have a multiple-choice and then hit “Submit” on the bottom. As everyone fills that out, if you would, if you’re interested, if we get 40 people typing the word “poll” in the chat box, if you chat it to everyone… Try not to just chat it to the speakers, hosts, and panelists. Go ahead and type the word “poll”. If we get 40, we’ll share all the poll results from today’s session with those who have submitted the information. We’re just looking for 40. But go ahead and type those in. And make sure you select everyone so that there’s more visibility and it’s easier to count. Entering this question is harder for Ky to add up, but she’s quite capable of that. So that would be supervised. It wouldn’t be a headless process that you’d be using, but… Go ahead, complete that. And then, just by way of guidance, Joseph, we’ll probably step over the system integration and skip over the initial at scale, the actual packet of requirements to support the data mindset. Just from a time perspective and the fact that we’ve covered some of these areas pretty well, I think that’ll keep it tight. But let’s go ahead and share the results. And thanks, everybody, for responding! The biggest challenge, insufficient data access. Interesting! And about a quarter, the biggest issue are the excessive amounts of data. Really, really interesting to me! Yeah, concepts of technology will shift the treasury’s paradigm. This is a good group for you and your company, Joseph.
Joseph Drambarean: As we’re talking through all of these different concepts today, I think what we’re kind of getting to as a conclusion is that there is a shift. There’s a shift of mindset. And I know that we’re gonna touch on that a little bit. But it’s interesting to see that we have a very self-aware group.
Craig Jeffery: Yeah, so in this slide, really, there’s the concept that some of these tools, whether it’s Twitter, Facebook, etc., serve us massive amounts of data and require a performance environment not necessarily to scale treasury needs. But there are some concepts there. We’re gonna pass over those lightly. And then, here, Joseph, I don’t know if you had an elevator pitch on the integration, how things change from passing data via email to asynchronous, bisynchronous, to a portal and file-based, and some of the summary items. Anything that you wanted to highlight because you’ve covered a number of good parts? Is there anything you wanted to call out before we move on?
Joseph Drambarean: Some of the more interesting pieces here are the developments because I remember there was a question earlier on, “Why would we go off of Swift to modern infrastructure?” Like you said, super complex answer. But one interesting anecdote to draw from this slide is that, as banks add new technology capabilities, whether it’s new reporting formats or new information data types, maybe more context from remittance details and things of that nature, they will not push those resources to legacy formats. It will be new. It will be available through API. And you will only be able to take advantage of it through API. One really great example of that right now is RTP. RTP is a payment technology that as of right now is not made available through the traditional banking portal on every single bank. However, it is API first, meaning that if you have an internal capacity for being able to use APIs and being able to take advantage of these types of systems, or you have a partner that you’re working with that can nimbly react to these types of technologies, you get a leg up, you have more advanced capabilities. And these capabilities can be onboarded and drive value immediately, as opposed to waiting what could be years for legacy providers or older solutions to onboard that capability. And it comes down to how you structure your technology stack: instead of focusing on purchasing applications or purchasing a solution, focusing instead on platform, focusing on how you can use a scale platform for driving a variety of use cases for a variety of stakeholders and really future proof your needs from a technology perspective for the next 10 years, not for the next 1.5 years of reporting that you need to do. And that’s a completely different mindset.
Craig Jeffery: Yeah! Yeah, thanks! And the concept of the requirement to support the data mindset. You’ve touched on a lot of these. I think about I Love Lucy when she’s working at, I don’t know, if it’s a candy factory, and she gets behind, there’s too much chocolate coming just like there might be too much data, and she’s sticking stuff in her mouth. That may be one of the challenges. Any summary thoughts to emphasize on this concept about what won’t work in the old environment or what’s required for the new environment?
Joseph Drambarean: I think that one thing that is interesting, that we probably haven’t touched on is, “What does the future of banking look like? And ultimately, will your choice of partners actually help play into the decisions that you make from a data perspective, how that data is stored, what the data is used for, the technology that is accessing that data, the applications downstream that need that data for processing, using the ML approaches that we talked about before? If we really believe the thesis of this talk that it starts with the data, which ultimately means it starts with your provider… It’s kind of the most basic form of the question. And I think that it’s gonna drive a change in how we shop for partners both from a technology perspective, but also from a banking perspective. And it can drive real value, especially when, ultimately, the insights and the decisions that are driven based on that data translate to potentially hundreds of thousands of dollars in savings in operational efficiency or maybe even a business strategy that could be millions worth, ultimately, when it comes down to it.
Craig Jeffery: Yeah, you’ve made a bunch of good points. I’m gonna comment on a couple of your points when we pull up our final poll question. And just so you know, we did get enough people typing in the word “poll”. So those will be sent out to you. Go ahead and select all that apply. To your point, Joseph, that people will make decisions based upon what can their technology provider do, what can their bank do, etc., we see that with people making decisions based upon, “Are you supporting better payment methods? I’m willing to move banks. I’m willing to move to banks that aren’t in my credit facility. Issues with KYC access to data. I’m willing to move and prioritize banks that support that activity.” So that is true.
One of the questions came up, “Is cloud data affecting the way treasury work, or is it just noise?” Two or three years ago, I’d say it was much more early noise on the horizon, maybe background radiation in the universe. It’s definitely impacting the way treasury is working. And it’s coming in faster than people think it will. In other words, they say, “We’re going to adopt this” or “We’re looking at this. We look at the percentages out a couple of years.” And when we’ve looked at a one-year time frame, those that have adopted it exceeded what the expectation was in two years. So those are a couple of the points there.
But let’s go ahead and look at the poll responses just real brief. Here, as we said, we’re gonna send these to you. We’re gonna close out that poll. We’re going to move ahead, Joseph, because I really wanna hear your thoughts. This is the context of the impact of treasury: liquidity cash flow, analysis, and insights. But I’d really rather you talk about that in conjunction with some of the final thoughts. How does treasury need to think? What are the blind spots? What do I need to be thinking about from a mindset perspective, from a data and a tech perspective? And how are you recommending people move ahead in this environment?
Joseph Drambarean: Yeah, I think that there are talking on the strengths that we’ve been talking about so far with regards to this shift, this transformation of not only the role, but the approach, and ultimately the technology that drives it. What we’re seeing is generally a move to two disparate types of interplay. The one is management and governance of banking relationships, and how they drive value not only to treasury but to the rest of financial operations within your company. And depending on the mix of the company, the size of the company, the complexity of the company, that could mean very impactful things, whether it’s downstream to accounting, whether it’s downstream to financial planning, whether it’s at the C-suite level. All of those different groups rely on core data coming from bank transactions and ultimately records of truth with regards to the banking relationships that you might have.
Now, specifically for treasury, organizing that data in the short term and driving value is really around the insights that you need to operate efficiently and make your business decisions with regards to liquidity. And that really could be a very short-term objective that has massive long-term implications if done with a data mindset, a data transformation mindset specifically. And that shift in terms of strategy, when you think about it, at the surface level is follow our checklist, taking these things into consideration that we’ve said. But at a more nuanced and macro level, it’s actually a transformation of the role, our role to be more technology-driven, more focused on the capabilities of technology, and ultimately be aware and driven to ultimately enforce and drive technology strategy within the finance organization when it relates to banking relationships and other kind of metadata related to those banking relationships. And that kind of strategy posture will drive decisions within vendor acquisition and technology acquisition, technology infrastructure, and how it plays into the needs that you will have both within treasury, but also within the wider universe of finance operations. The stakeholders that need this data and depend on it for accuracy, depend on it for real-time capabilities and, ultimately, then the ML capabilities that will be driven, that ultimately has to be considered upfront, and it creates this shift in the role. That creates almost like a morphed role that is both treasury, both technology-driven in one role and has a tremendous amount of long-term leadership when you think about it from an organizational perspective.
And that’s how we’ve been thinking about this, that it’s not just the short-term play. It’s a long-term play. And when accepted as such, it’ll drive choices when it comes to banking relationships. It’ll drive choices when it comes to technology both from a foundations perspective and from an end vendor and application perspective. It’ll drive organizational governance. One obvious example when thinking about the fragmentation of bank data within the enterprise is, “Who needs bank transactions and for what? And which set are they looking at? And can you guarantee that what they’re looking at is accurate?” That kind of governance at a meta-level is something that we have seen best suited to treasury. And treasury sits in an optimal position when it comes to relationships with the banks, relationships with the stakeholders to be able to drive that kind of value. So I think that when you look at it holistically, yes, it starts with better insights, faster reporting, better gap filling when it comes to the treasury role. But when you go full lifecycle on that flywheel that we showed in the previous slide, it really expands to be a more meta role and a more meta mindset.
Craig Jeffery: Yeah, Joseph, it’s been fun talking with you on this topic on tech, how it’s impacting treasury, how these things relate. It’s also been good hearing a bunch of terms you don’t always hear in treasuries much that are more talked about in the technologist arena. So everybody can use some of the terms like “headless”. That’s one I wanna use more frequently. I never think about that. But yeah, it’s been a great discussion. I wanted to also mention, there’s a comment about… Treasury is diving into order-to-cash and procure-to-pay at a deeper level to manage working capital. We also see this. This allows extensibility from liquidity to further into the cash conversion cycle, further into risk management and exposure management items. So all of these concepts that Joseph has been talking about continue to extend out to the world and the broader area of finance. And the cash conversion cycle is an interesting piece to that as well. So Joseph, thank you for your time and your comments! We have the next part in the series. It’s “Partnering with IT on Your Digital Transformation”, the fourth part, “Evaluating Tech Solution Sets”. This is part of this four-part, and perhaps more, Digital Transformation Strategy series. And with that, thank you, Joseph! And I’ll turn it back over to Ky, who’s been patient to bear with us hounding her about getting all of these poll questions answered. So Ky.
Ky: Thank you, everyone, for joining us today! The CPT credits, the webinar slides, and the recording of today’s webinar will be sent to you within five business days. We hope you’ll join us for part three of this webinar series “Partnering with IT on Your Digital Transformation” that will be held on September 14 at 2 pm Eastern. The registration link is in the chat box. We’re gonna stay online for a few more minutes if you have any questions. Otherwise, enjoy the rest of your day! Thanks for joining us!
Speaker
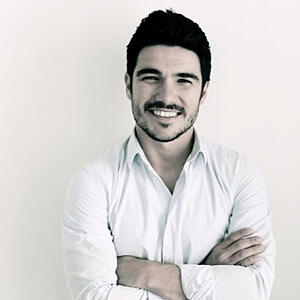
Working with key Fortune-level brands, including Capital One, Marriott International, Microsoft, Harley-Davidson, and Allstate Insurance, Joseph Drambarean has helped brands navigate the digital landscape by creating and executing innovative digital strategies, as well as enterprise product integrations that incorporate cloud architecture, analytical insights, industry-leading UI/UX, and technical recommendations designed to bring measurable ROI.